Spaces:
Sleeping
Sleeping
File size: 8,505 Bytes
2ba1947 e66a60a 2ba1947 e66a60a 2ba1947 e66a60a 2ba1947 e66a60a 2ba1947 e66a60a 2ba1947 e66a60a 2ba1947 e66a60a 2ba1947 e66a60a 2ba1947 e66a60a 2ba1947 e66a60a 2ba1947 e66a60a 2ba1947 e66a60a 2ba1947 e66a60a 2ba1947 e66a60a 2ba1947 e66a60a 2ba1947 e66a60a 2ba1947 e66a60a 2ba1947 e66a60a 2ba1947 e66a60a 2ba1947 e66a60a 2ba1947 e66a60a 2ba1947 e66a60a 2ba1947 |
1 2 3 4 5 6 7 8 9 10 11 12 13 14 15 16 17 18 19 20 21 22 23 24 25 26 27 28 29 30 31 32 33 34 35 36 37 38 39 40 41 42 43 44 45 46 47 48 49 50 51 52 53 54 55 56 57 58 59 60 61 62 63 64 65 66 67 68 69 70 71 72 73 74 75 76 77 78 79 80 81 82 83 84 85 86 87 88 89 90 91 92 93 94 95 96 97 98 99 100 101 102 103 104 105 106 107 108 109 110 111 112 113 114 115 116 117 118 119 120 121 122 123 124 125 126 127 128 129 130 131 132 133 134 135 136 137 138 139 140 141 142 143 144 145 146 147 148 149 150 151 152 153 154 155 156 157 158 159 160 161 162 163 164 165 166 167 168 169 170 171 172 173 174 175 176 177 178 179 180 181 182 183 184 185 186 187 188 189 190 191 192 193 194 195 196 197 198 199 200 201 202 203 204 205 206 207 208 209 210 211 212 213 214 215 216 217 218 219 220 221 222 223 224 225 226 227 228 229 230 231 232 233 234 235 236 237 238 239 240 241 242 243 244 245 246 247 248 249 250 251 252 253 254 255 256 257 258 259 260 261 262 263 264 265 266 267 268 269 270 271 272 |
{
"cells": [
{
"cell_type": "markdown",
"metadata": {},
"source": [
"<h1 align=center> Contextual RAG </h1>\n",
"\n",
"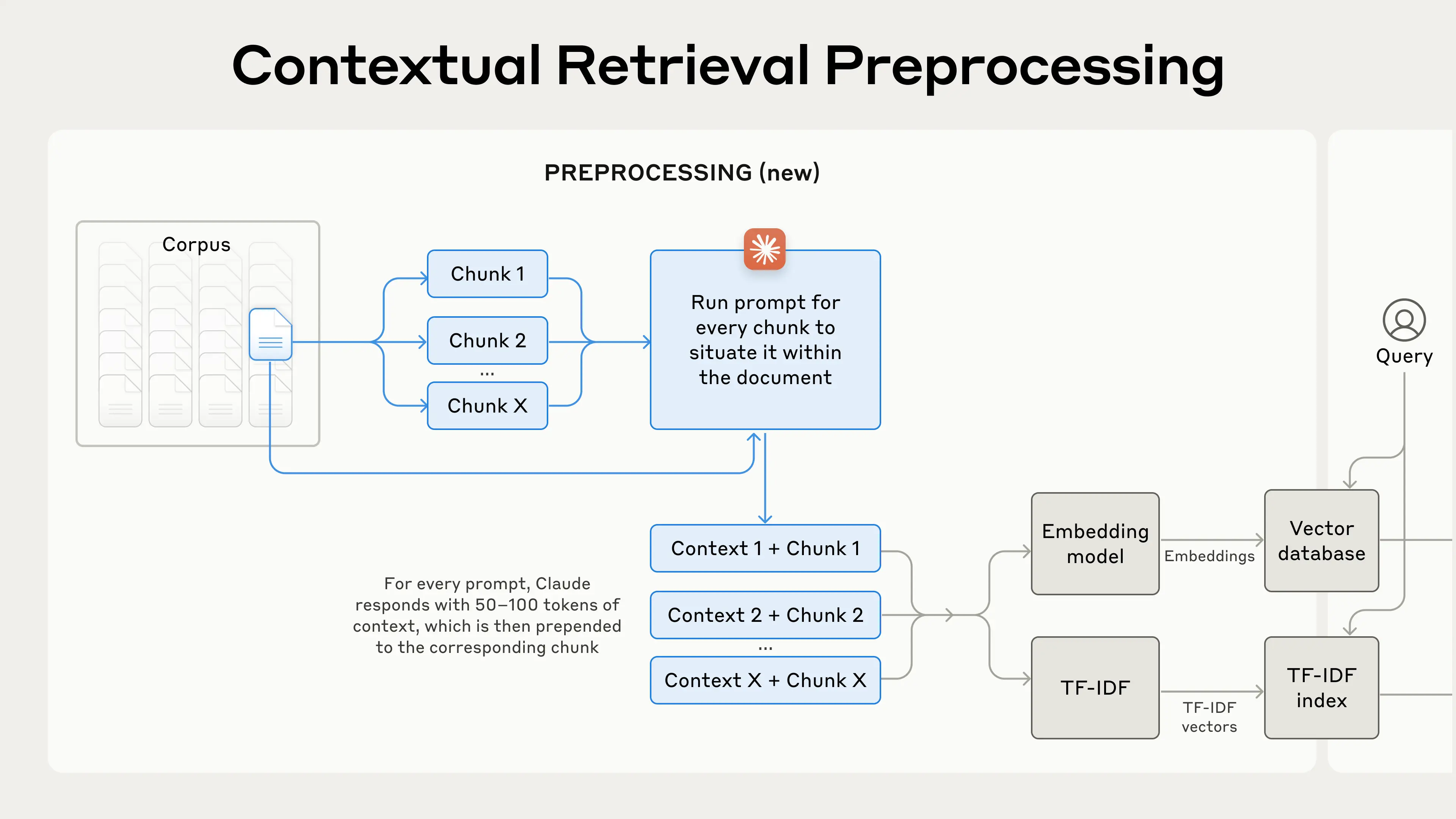\n",
"\n",
"This is an approach proposed by Anthropic in a recent [blog poas](https://www.anthropic.com/news/contextual-retrieval). It involves improving retrieval by providing each document chunk with an in context summary."
]
},
{
"cell_type": "markdown",
"metadata": {},
"source": [
"<h2 align=center> Problems </h2>\n",
"\n",
"As one may gather from the explanation, there is a requirement that each chunk be appropriately contextualized with respect to the rest of the document. So essentially the whole document has to be passed into the prompt each time along with the chunk. There are two problems with this:\n",
"\n",
"1. This would be very expensive in terms of input token count.\n",
"2. For models with smaller context windows, the whole document may exceed it.( Further, there is a sense in which fitting a whole document into a models context width defeats the point of performing RAG.)\n"
]
},
{
"cell_type": "markdown",
"metadata": {},
"source": [
"<h2 align=center> Whole Document Summarization </h2>\n",
"\n",
"The solution I have come up with is to instead summarize the document into a more manageable size."
]
},
{
"cell_type": "markdown",
"metadata": {},
"source": [
"<h3 align=center> Refine </h3>"
]
},
{
"cell_type": "code",
"execution_count": 1,
"metadata": {},
"outputs": [],
"source": [
"from langchain.chains.combine_documents.stuff import StuffDocumentsChain\n",
"from langchain.chains.llm import LLMChain\n",
"from langchain.prompts import PromptTemplate\n",
"from langchain_text_splitters import CharacterTextSplitter\n",
"from langchain.document_loaders import PyMuPDFLoader"
]
},
{
"cell_type": "code",
"execution_count": 2,
"metadata": {},
"outputs": [],
"source": [
"from langchain.chains.summarize import load_summarize_chain"
]
},
{
"cell_type": "code",
"execution_count": 3,
"metadata": {},
"outputs": [],
"source": [
"# from langchain_google_genai import ChatGoogleGenerativeAI\n",
"# import os\n",
"# from dotenv import load_dotenv\n",
"\n",
"# if not load_dotenv():\n",
"# print(\"API keys may not have been loaded succesfully\")\n",
"# google_api_key = os.getenv(\"GOOGLE_API_KEY\")\n",
"\n",
"# llm = ChatGoogleGenerativeAI(model=\"gemini-pro\", api_key=google_api_key)"
]
},
{
"cell_type": "code",
"execution_count": 4,
"metadata": {},
"outputs": [],
"source": [
"from langchain_ollama.llms import OllamaLLM\n",
"\n",
"# A lightweigh model for local inference\n",
"llm = OllamaLLM(model=\"llama3.2:1b-instruct-q4_K_M\")"
]
},
{
"cell_type": "code",
"execution_count": 5,
"metadata": {},
"outputs": [],
"source": [
"loader = PyMuPDFLoader(\"data/State Machines.pdf\")\n",
"docs = loader.load()"
]
},
{
"cell_type": "code",
"execution_count": 21,
"metadata": {},
"outputs": [],
"source": [
"text_splitter = CharacterTextSplitter.from_tiktoken_encoder(chunk_size=8000, chunk_overlap=0)\n",
"split_docs = text_splitter.split_documents(docs)"
]
},
{
"cell_type": "code",
"execution_count": null,
"metadata": {},
"outputs": [],
"source": [
"output_key = \"output_text\""
]
},
{
"cell_type": "code",
"execution_count": 22,
"metadata": {},
"outputs": [],
"source": [
"prompt = \"\"\"\n",
" Please provide a very comprehensive summary of the following text.\n",
" WHile maintaining lower level detail\n",
" \n",
" TEXT: {text}\n",
" SUMMARY:\n",
" \"\"\"\n",
"\n",
"question_prompt = PromptTemplate(\n",
" template=prompt, input_variables=[\"text\"]\n",
")\n",
"\n",
"refine_prompt_template = \"\"\"\n",
" Write a comprehensive summary of the following text delimited by triple backquotes.\n",
" Your goal will be to give a high level overview while also expounding on some finer details of the text\n",
"\n",
" ```{text}```\n",
" \n",
" Have your answer in about 1500 words\n",
" \"\"\"\n",
"\n",
"\n",
"refine_template = PromptTemplate(\n",
" template=refine_prompt_template, input_variables=[\"text\"]\n",
")\n",
"\n",
"# Load refine chain\n",
"chain = load_summarize_chain(\n",
" llm=llm,\n",
" chain_type=\"refine\",\n",
" question_prompt=question_prompt,\n",
" refine_prompt=refine_template,\n",
" return_intermediate_steps=True,\n",
" input_key=\"input_documents\",\n",
" output_key=output_key,\n",
")\n",
"result = chain({\"input_documents\": split_docs}, return_only_outputs=True)"
]
},
{
"cell_type": "code",
"execution_count": 23,
"metadata": {},
"outputs": [],
"source": [
"from IPython.display import display, Markdown"
]
},
{
"cell_type": "code",
"execution_count": 24,
"metadata": {},
"outputs": [
{
"data": {
"text/markdown": [
"Here is a summary of the text:\n",
"\n",
"A state machine is a mathematical model that describes how an output signal is generated from an input signal step-by-step. It consists of five main components: \n",
"\n",
"1. States (representing different states or conditions)\n",
"2. Inputs (input signals, such as letters or symbols)\n",
"3. Outputs (output signals, which represent the actual output based on the input and state)\n",
"4. Update function (a way to modify the current state based on the inputs and outputs)\n",
"5. Initial State (the starting point of the machine)\n",
"\n",
"An example is given where a state machine is defined with three states: States, Inputs, Outputs. The initial state is also provided as an option.\n",
"\n",
"The key points are:\n",
"\n",
"* Time is not involved in this model; instead, step numbers refer to the order in which steps occur.\n",
"* Each input signal can be represented by an infinite sequence of symbols, such as a natural number sequence (e.g., 0 -> Inputs).\n",
"* The state machine evolves or \"moves\" from one state to another based on the inputs and outputs.\n",
"\n",
"This model is used for various applications, including control systems, data processing, and communication systems."
],
"text/plain": [
"<IPython.core.display.Markdown object>"
]
},
"metadata": {},
"output_type": "display_data"
}
],
"source": [
"display(Markdown(result[output_key]))"
]
},
{
"cell_type": "code",
"execution_count": null,
"metadata": {},
"outputs": [],
"source": []
},
{
"cell_type": "markdown",
"metadata": {},
"source": [
"<h3 align=center> Remarks </h3>\n",
"\n",
"Refine is properly configured but we ran into this error.\n",
"\n",
"```python\n",
"ResourceExhausted: 429 Resource has been exhausted (e.g. check quota).\n",
"```\n",
"\n",
"This is a problem on the part of our llm provider not the code.\n",
"\n",
"<h3 align=center> Next Steps </h3>\n",
"\n",
"The best approach will be to use local models to achive this kind of heavy inference. For that we will turn to either **Ollama** or hugging face **Transformers**."
]
},
{
"cell_type": "code",
"execution_count": null,
"metadata": {},
"outputs": [],
"source": []
}
],
"metadata": {
"kernelspec": {
"display_name": ".venv",
"language": "python",
"name": "python3"
},
"language_info": {
"codemirror_mode": {
"name": "ipython",
"version": 3
},
"file_extension": ".py",
"mimetype": "text/x-python",
"name": "python",
"nbconvert_exporter": "python",
"pygments_lexer": "ipython3",
"version": "3.10.12"
}
},
"nbformat": 4,
"nbformat_minor": 2
}
|