FRACTAL-LidarHD_7cl_randlanet
The general characteristics of this specific model FRACTAL-LidarHD_7cl_randlanet are :
- Trained with the FRACTAL dataset for the semantic segmentation of Lidar HD point clouds
- Aerial lidar point clouds, colorized with rgb + near-infrared, with high point density (~40 pts/m²)
- RandLa-Net architecture as implemented in the Myria3D library
- 7 class nomenclature : other, ground, vegetation, building, water, bridge, permanent structure
Model Informations
- Code repository: https://github.com/IGNF/myria3d (V3.8)
- Paper: TBD
- Developed by: IGN
- Compute infrastructure:
- software: python, pytorch-lightning
- hardware: in-house HPC/AI resources
- License: : Etalab 2.0
Uses
The model was specifically trained for the semantic segmentation of aerial lidar point clouds from the Lidar HD program (2020-2025).
Aerial Lidar scene understanding: the model is designed for the segmentation of aerial lidar point clouds into 7 classes: other | ground | vegetation | building | water | bridge | permanent structure. While the model could be applied to other types of point clouds (mobile, terrestrial), aerial lidar scanning has specific geometric specifications (occlusions, homogeneous densities, variable scanner angle...). Furthermore, the aerial images used for point cloud colorization (from the (BD ORTHO®), have their own spatial and radiometric specifications. Therefore, the model is best optimized for aerial lidar point clouds with similar densities and colorimetries than the original ones.
Bias, Risks, Limitations and Recommendations
Spatial Generalization: The FRACTAL dataset used for training covers 5 spatial domains from 5 southern regions of metropolitan France. While large and diverse, the dataset covers only a fraction of the French territory, and are not representative of its full diversity (landscapes, hardscapes, human-made objects...). Adequate verifications and evaluations should be done when applied to new spatial domains.
Using the model for other data sources: The model was trained on Lidar HD data that was colorized with very high resolution aerial images from the ORTHO HR database. The data sources have their specificities in terms of resolution and spectral domains. Users can expect a drop in performance with other 3D and 2D data sources. This being said, while domain shifts are frequent for aerial imageries due to different acquisition conditions and downstream data processing, aerial lidar point clouds of comparable point densities (~40 pts/m²) are expected to have more consistent geometric characteristiques across spatial domains.
How to Get Started with the Model
Model was trained in an open source deep learning code repository developped in-house: github.com/IGNF/myria3d). Inference is only supported in this library, and inference instructions are detailed in the code repository documentation. Patched inference from large point clouds (e.g. 1 x 1 km Lidar HD tiles) is supported, with or without (by default) overlapping sliding windows. The original point cloud is augmented with several dimensions: a PredictedClassification dimension, an entropy dimension, and (optionnaly) class probability dimensions (e.g. building, ground...). For convenience and scalable model deployment, Myria3D comes with a Dockerfile.
Training Details
The data comes from the Lidar HD program, more specifically from acquisition areas that underwent automated classification followed by manual correction (so-called "optimized Lidar HD"). It meets the quality requirements of the Lidar HD program, which accepts a controlled level of classification errors for each semantic class. The model was trained on FRACTAL, a benchmark dataset for semantic segmentation. FRACTAL contains 250 km² of data sampled from an original 17440 km² area, with a large diversity of landscapes and scenes.
Training Data
80,000 point cloud patches of 50 x 50 meters each (200 km²) were used to train the FRACTAL-LidarHD_7cl_randlanet model. 10,000 additional patches (25 km²) were used for model validation.
Training Procedure
Preprocessing
Point clouds were preprocessed for training with point subsampling, filtering of artefacts points, on-the-fly creation of colorimetric features, and normalization of features and coordinates. For inference, a preprocessing as close as possible should be used. Refer to the inference configuration file, and to the Myria3D code repository (V3.8).
Training Hyperparameters
- Model architecture: RandLa-Net (implemented with the Pytorch-Geometric framework in [Myria3D](https://github.com/IGNF/myria3d/blob/main/myria3d/models/modules/pyg_randla_net.py))
- Augmentation :
- VerticalFlip(p=0.5)
- HorizontalFlip(p=0.5)
- Features:
- Lidar: x, y, z, echo number (1-based numbering), number of echos, reflectance (a.k.a intensity)
- Colors:
- Original: RGB + Near-Infrared (colorization from aerial images by vertical pixel-point alignement)
- Derived: average color = (R+G+B)/3 and NDVI.
- Input preprocessing:
- grid sampling: 0.25 m
- random sampling: 40,000 (if higher)
- horizontal normalization: mean xy substraction
- vertical normalization: min z substraction
- coordinates normalization: division by 25 meters
- basic occlusion model: nullify color channels if echo_number > 1
- features scaling (0-1 range):
- echo number and number of echos: division by 7
- color (r, g, b, near-infrared, average color): division by 65280 (i.e. 255*256)
- features normalization:
- reflectance: log-normalization, standardization, clipping of amplitude above 3 standard deviations.
- average color: same as reflectance.
- Batch size: 10 (x 6 GPUs)
- Number of epochs : 100 (min) - 150 (max)
- Early stopping : patience 6 and val_loss as monitor criterium
- Loss: Cross-Entropy
- Optimizer : Adam
- Scheduler : mode = "min", factor = 0.5, patience = 20, cooldown = 5
- Learning rate : 0.004
Speeds, Sizes, Times
The FRACTAL-LidarHD_7cl_randlanet model was trained on an in-house HPC cluster. 6 V100 GPUs were used (2 nodes, 3 GPUS per node). With this configuration the approximate learning time is 30 minutes per epoch. The model was obtained for num_epoch=21 with corresponding val_loss=0.112.
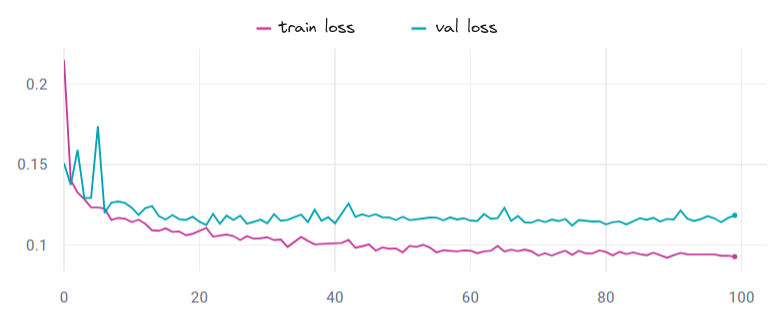
Evaluation
Testing Data, Factors & Metrics
Testing Data
The model was evaluated on the 10,000 data patches of the test set of the FRACTAL dataset, that are independant from train and val patches, and sampled from distinct areas in the five spatial domains of the dataset. The diversity of landscapes and scenes of the test set should closely match the one of the train and val sets.
Metrics
The FRACTAL-LidarHD_7cl_randlanet model achieves a performance of mIoU=77.2% and OA=96.1%.
The following table gives the class-wise metrics on the test set:
Class | IoU | Accuracy | Precision | Recall | F1 |
---|---|---|---|---|---|
other | 47.5 | 54.9 | 77.8 | 54.9 | 64.4 |
ground | 91.9 | 97.7 | 93.8 | 97.7 | 95.8 |
vegetation | 93.8 | 95.6 | 98.0 | 95.6 | 96.8 |
building | 90.4 | 93.7 | 96.2 | 93.7 | 95.0 |
water | 90.1 | 92.6 | 97.1 | 92.6 | 94.8 |
bridge | 65.2 | 78.6 | 79.3 | 78.6 | 79.0 |
permanent structure | 63.5 | 76.6 | 78.9 | 76.6 | 77.7 |
Macro Average | 77.5 | 84.2 | 88.7 | 84.2 | 86.2 |
The following illustration gives the resulting confusion matrix :
- Left : normalised acording to rows: rows sum at 100% and the recall is on the diagonal of the matrix
- Right : normalised acording to columns: columns sum at 100% and the precision is on the diagonal of the matrix
Normalized Confusion Matrices. (a) Recall, (b) Precision)
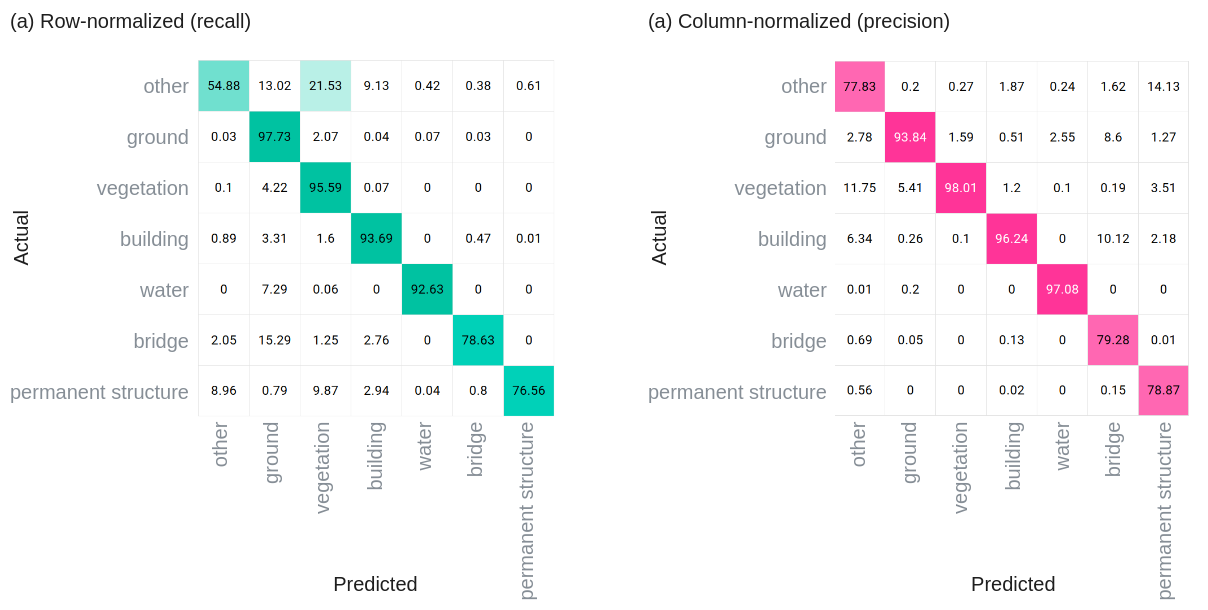
Results
From test patches with at least 10k points (i.e. at least 4 pts/m²), we sample patches without cherry-picking, to match matches with the following metadata: a) URBAN, b) WATER & BRIDGE, c) OTHER_PARKING, d) BUILD_GREENHOUSE, e) HIGHSLOPE.
Input point cloud, target classification, and model prediction for a subset of patches from the test set of FRACTAL.

Citation
BibTeX:
@misc{gaydon2024fractal,
title={FRACTAL: An Ultra-Large-Scale Aerial Lidar Dataset for 3D Semantic Segmentation of Diverse Landscapes},
author={Charles Gaydon and Michel Daab and Floryne Roche},
year={2024},
eprint={TBD},
archivePrefix={arXiv},
url={https://arxiv.org/abs/TBD}
primaryClass={cs.CV}
}
Contact : TBD
Evaluation results
- mIoU on IGNF/FRACTALself-reported77.500
- IoU Other on IGNF/FRACTALself-reported47.500
- IoU Ground on IGNF/FRACTALself-reported91.900
- IoU Vegetation on IGNF/FRACTALself-reported93.800
- IoU Building on IGNF/FRACTALself-reported90.400
- IoU Water on IGNF/FRACTALself-reported90.100
- IoU Bridge on IGNF/FRACTALself-reported65.200
- IoU Permanent Structure on IGNF/FRACTALself-reported63.500
- mIoU on eval67 (secret test set)self-reported60.800
- IoU Other on eval67 (secret test set)self-reported22.300