The text embedding set trained by Jina AI.
Quick Start
The easiest way to starting using jina-embeddings-v2-small-en
is to use Jina AI's Embedding API.
Intended Usage & Model Info
jina-embeddings-v2-small-en
is an English, monolingual embedding model supporting 8192 sequence length.
It is based on a BERT architecture (JinaBERT) that supports the symmetric bidirectional variant of ALiBi to allow longer sequence length.
The backbone jina-bert-v2-small-en
is pretrained on the C4 dataset.
The model is further trained on Jina AI's collection of more than 400 millions of sentence pairs and hard negatives.
These pairs were obtained from various domains and were carefully selected through a thorough cleaning process.
The embedding model was trained using 512 sequence length, but extrapolates to 8k sequence length (or even longer) thanks to ALiBi. This makes our model useful for a range of use cases, especially when processing long documents is needed, including long document retrieval, semantic textual similarity, text reranking, recommendation, RAG and LLM-based generative search, etc.
This model has 33 million parameters, which enables lightning-fast and memory efficient inference, while still delivering impressive performance. Additionally, we provide the following embedding models:
jina-embeddings-v2-small-en
: 33 million parameters (you are here).jina-embeddings-v2-base-en
: 137 million parameters.jina-embeddings-v2-base-zh
: 161 million parameters Chinese-English Bilingual embeddings.jina-embeddings-v2-base-de
: 161 million parameters German-English Bilingual embeddings.jina-embeddings-v2-base-es
: Spanish-English Bilingual embeddings (soon).
Data & Parameters
Jina Embeddings V2 technical report
Usage
Please apply mean pooling when integrating the model.
Why mean pooling?
mean poooling
takes all token embeddings from model output and averaging them at sentence/paragraph level.
It has been proved to be the most effective way to produce high-quality sentence embeddings.
We offer an encode
function to deal with this.
However, if you would like to do it without using the default encode
function:
import torch
import torch.nn.functional as F
from transformers import AutoTokenizer, AutoModel
def mean_pooling(model_output, attention_mask):
token_embeddings = model_output[0]
input_mask_expanded = attention_mask.unsqueeze(-1).expand(token_embeddings.size()).float()
return torch.sum(token_embeddings * input_mask_expanded, 1) / torch.clamp(input_mask_expanded.sum(1), min=1e-9)
sentences = ['How is the weather today?', 'What is the current weather like today?']
tokenizer = AutoTokenizer.from_pretrained('jinaai/jina-embeddings-v2-small-en')
model = AutoModel.from_pretrained('jinaai/jina-embeddings-v2-small-en', trust_remote_code=True)
encoded_input = tokenizer(sentences, padding=True, truncation=True, return_tensors='pt')
with torch.no_grad():
model_output = model(**encoded_input)
embeddings = mean_pooling(model_output, encoded_input['attention_mask'])
embeddings = F.normalize(embeddings, p=2, dim=1)
You can use Jina Embedding models directly from transformers package.
!pip install transformers
from transformers import AutoModel
from numpy.linalg import norm
cos_sim = lambda a,b: (a @ b.T) / (norm(a)*norm(b))
model = AutoModel.from_pretrained('jinaai/jina-embeddings-v2-small-en', trust_remote_code=True) # trust_remote_code is needed to use the encode method
embeddings = model.encode(['How is the weather today?', 'What is the current weather like today?'])
print(cos_sim(embeddings[0], embeddings[1]))
If you only want to handle shorter sequence, such as 2k, pass the max_length
parameter to the encode
function:
embeddings = model.encode(
['Very long ... document'],
max_length=2048
)
The latest sentence-transformers also supports Jina embeddings:
!pip install -U sentence-transformers
from sentence_transformers import SentenceTransformer
from sentence_transformers.util import cos_sim
model = SentenceTransformer(
"jinaai/jina-embeddings-v2-small-en", # switch to en/zh for English or Chinese
trust_remote_code=True
)
# control your input sequence length up to 8192
model.max_seq_length = 1024
embeddings = model.encode([
'How is the weather today?',
'What is the current weather like today?'
])
print(cos_sim(embeddings[0], embeddings[1]))
Alternatives to Using Transformers Package
- Managed SaaS: Get started with a free key on Jina AI's Embedding API.
- Private and high-performance deployment: Get started by picking from our suite of models and deploy them on AWS Sagemaker.
RAG Performance
According to the latest blog post from LLamaIndex,
In summary, to achieve the peak performance in both hit rate and MRR, the combination of OpenAI or JinaAI-Base embeddings with the CohereRerank/bge-reranker-large reranker stands out.
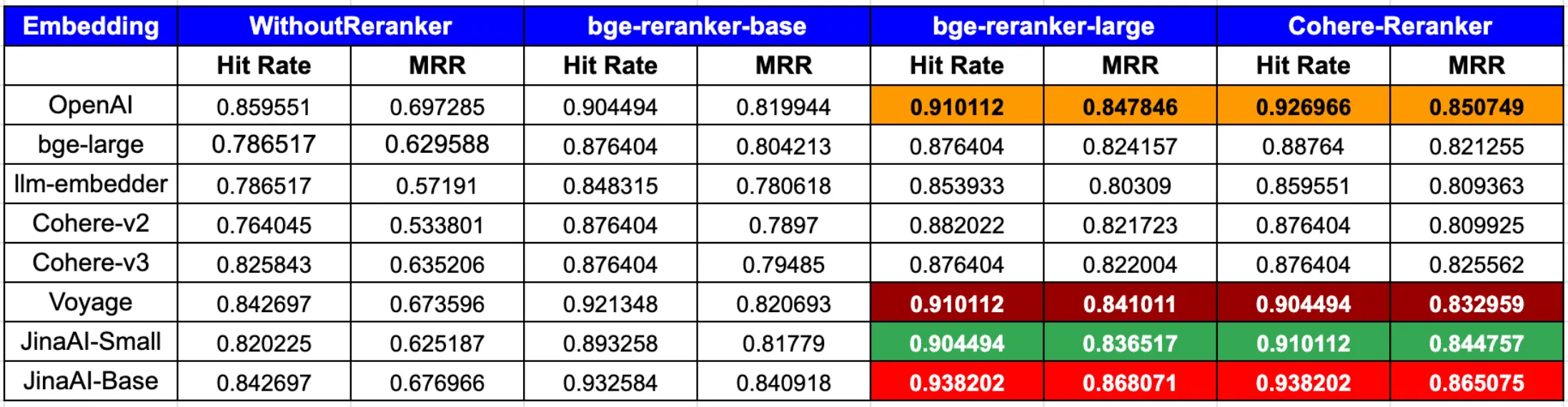
Plans
- Bilingual embedding models supporting more European & Asian languages, including Spanish, French, Italian and Japanese.
- Multimodal embedding models enable Multimodal RAG applications.
- High-performt rerankers.
Trouble Shooting
Loading of Model Code failed
If you forgot to pass the trust_remote_code=True
flag when calling AutoModel.from_pretrained
or initializing the model via the SentenceTransformer
class, you will receive an error that the model weights could not be initialized.
This is caused by tranformers falling back to creating a default BERT model, instead of a jina-embedding model:
Some weights of the model checkpoint at jinaai/jina-embeddings-v2-base-en were not used when initializing BertModel: ['encoder.layer.2.mlp.layernorm.weight', 'encoder.layer.3.mlp.layernorm.weight', 'encoder.layer.10.mlp.wo.bias', 'encoder.layer.5.mlp.wo.bias', 'encoder.layer.2.mlp.layernorm.bias', 'encoder.layer.1.mlp.gated_layers.weight', 'encoder.layer.5.mlp.gated_layers.weight', 'encoder.layer.8.mlp.layernorm.bias', ...
Contact
Join our Discord community and chat with other community members about ideas.
Citation
If you find Jina Embeddings useful in your research, please cite the following paper:
@misc{günther2023jina,
title={Jina Embeddings 2: 8192-Token General-Purpose Text Embeddings for Long Documents},
author={Michael Günther and Jackmin Ong and Isabelle Mohr and Alaeddine Abdessalem and Tanguy Abel and Mohammad Kalim Akram and Susana Guzman and Georgios Mastrapas and Saba Sturua and Bo Wang and Maximilian Werk and Nan Wang and Han Xiao},
year={2023},
eprint={2310.19923},
archivePrefix={arXiv},
primaryClass={cs.CL}
}
- Downloads last month
- 409,197
Model tree for jinaai/jina-embeddings-v2-small-en
Dataset used to train jinaai/jina-embeddings-v2-small-en
Spaces using jinaai/jina-embeddings-v2-small-en 5
Collection including jinaai/jina-embeddings-v2-small-en
Evaluation results
- accuracy on MTEB AmazonCounterfactualClassification (en)test set self-reported71.358
- ap on MTEB AmazonCounterfactualClassification (en)test set self-reported33.999
- f1 on MTEB AmazonCounterfactualClassification (en)test set self-reported65.385
- accuracy on MTEB AmazonPolarityClassificationtest set self-reported82.901
- ap on MTEB AmazonPolarityClassificationtest set self-reported78.014
- f1 on MTEB AmazonPolarityClassificationtest set self-reported82.834
- accuracy on MTEB AmazonReviewsClassification (en)test set self-reported40.890
- f1 on MTEB AmazonReviewsClassification (en)test set self-reported39.209
- map_at_1 on MTEB ArguAnatest set self-reported23.257
- map_at_10 on MTEB ArguAnatest set self-reported37.946