File size: 8,069 Bytes
440604d d9d82d2 440604d 37ed39e 440604d ce22a9f 440604d 37ed39e 440604d 53a0ca3 440604d fb266b3 440604d fb266b3 440604d 37ed39e 440604d 37ed39e 440604d 37ed39e 440604d 37ed39e 440604d 92f6500 440604d |
1 2 3 4 5 6 7 8 9 10 11 12 13 14 15 16 17 18 19 20 21 22 23 24 25 26 27 28 29 30 31 32 33 34 35 36 37 38 39 40 41 42 43 44 45 46 47 48 49 50 51 52 53 54 55 56 57 58 59 60 61 62 63 64 65 66 67 68 69 70 71 72 73 74 75 76 77 78 79 80 81 82 83 84 85 86 87 88 89 90 91 92 93 94 95 96 97 98 99 100 101 102 103 104 105 106 107 108 109 110 111 112 113 114 115 116 117 118 119 120 121 122 123 124 125 126 127 128 129 130 131 132 133 134 135 136 137 138 139 140 141 142 143 144 145 146 147 148 149 150 151 152 153 154 155 156 157 158 159 160 161 162 163 |
---
datasets:
- cityscapes
library_name: pytorch
license: bsd-3-clause
pipeline_tag: image-segmentation
tags:
- quantized
- real_time
- android
---
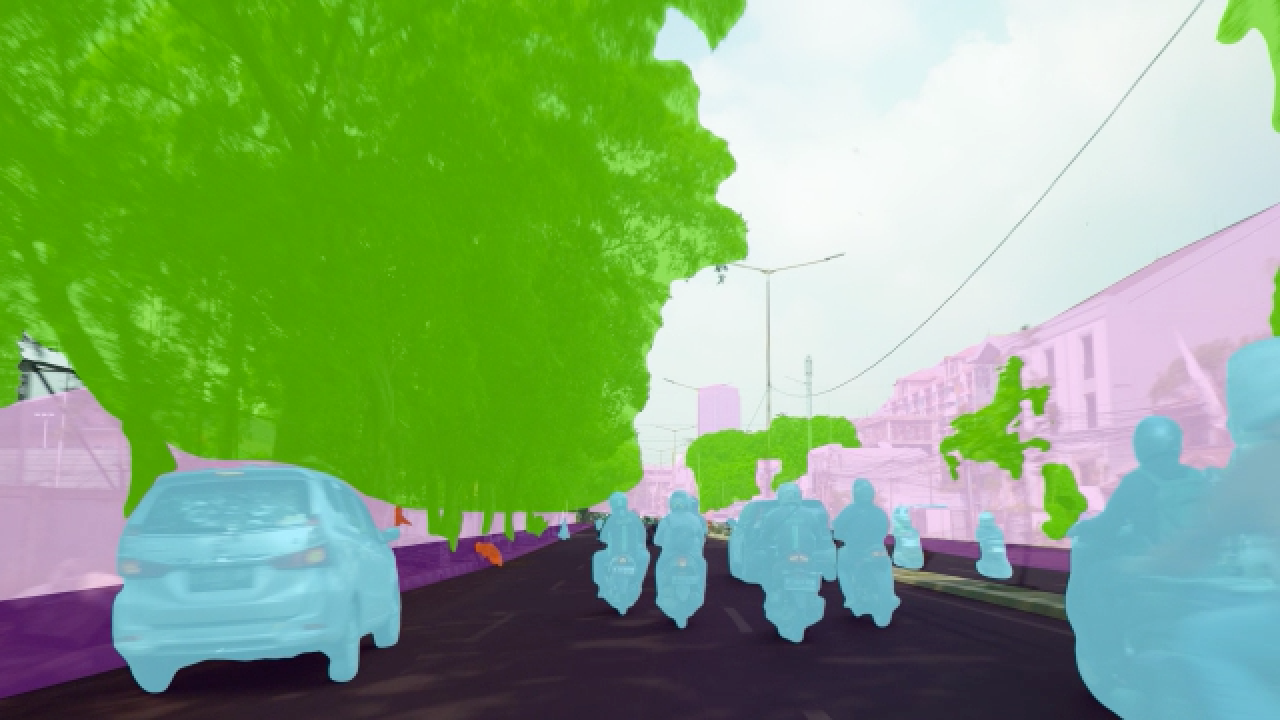
# FFNet-78S-Quantized: Optimized for Mobile Deployment
## Semantic segmentation for automotive street scenes
FFNet-78S-Quantized is a "fuss-free network" that segments street scene images with per-pixel classes like road, sidewalk, and pedestrian. Trained on the Cityscapes dataset.
This model is an implementation of FFNet-78S-Quantized found [here]({source_repo}).
This repository provides scripts to run FFNet-78S-Quantized on Qualcomm® devices.
More details on model performance across various devices, can be found
[here](https://aihub.qualcomm.com/models/ffnet_78s_quantized).
### Model Details
- **Model Type:** Semantic segmentation
- **Model Stats:**
- Model checkpoint: ffnet78S_dBBB_cityscapes_state_dict_quarts
- Input resolution: 2048x1024
- Number of parameters: 27.5M
- Model size: 26.7 MB
- Number of output classes: 19
| Model | Device | Chipset | Target Runtime | Inference Time (ms) | Peak Memory Range (MB) | Precision | Primary Compute Unit | Target Model
|---|---|---|---|---|---|---|---|---|
| FFNet-78S-Quantized | Samsung Galaxy S23 | Snapdragon® 8 Gen 2 | TFLITE | 5.745 ms | 0 - 2 MB | INT8 | NPU | [FFNet-78S-Quantized.tflite](https://huggingface.co/qualcomm/FFNet-78S-Quantized/blob/main/FFNet-78S-Quantized.tflite) |
| FFNet-78S-Quantized | Samsung Galaxy S23 | Snapdragon® 8 Gen 2 | ONNX | 11.963 ms | 0 - 24 MB | INT8 | NPU | [FFNet-78S-Quantized.onnx](https://huggingface.co/qualcomm/FFNet-78S-Quantized/blob/main/FFNet-78S-Quantized.onnx) |
| FFNet-78S-Quantized | Samsung Galaxy S24 | Snapdragon® 8 Gen 3 | TFLITE | 4.089 ms | 1 - 86 MB | INT8 | NPU | [FFNet-78S-Quantized.tflite](https://huggingface.co/qualcomm/FFNet-78S-Quantized/blob/main/FFNet-78S-Quantized.tflite) |
| FFNet-78S-Quantized | Samsung Galaxy S24 | Snapdragon® 8 Gen 3 | ONNX | 8.58 ms | 5 - 151 MB | INT8 | NPU | [FFNet-78S-Quantized.onnx](https://huggingface.co/qualcomm/FFNet-78S-Quantized/blob/main/FFNet-78S-Quantized.onnx) |
| FFNet-78S-Quantized | RB3 Gen 2 (Proxy) | QCS6490 Proxy | TFLITE | 35.597 ms | 1 - 48 MB | INT8 | NPU | [FFNet-78S-Quantized.tflite](https://huggingface.co/qualcomm/FFNet-78S-Quantized/blob/main/FFNet-78S-Quantized.tflite) |
| FFNet-78S-Quantized | RB5 (Proxy) | QCS8250 Proxy | TFLITE | 218.427 ms | 1 - 3 MB | INT8 | NPU | [FFNet-78S-Quantized.tflite](https://huggingface.co/qualcomm/FFNet-78S-Quantized/blob/main/FFNet-78S-Quantized.tflite) |
| FFNet-78S-Quantized | QCS8550 (Proxy) | QCS8550 Proxy | TFLITE | 5.683 ms | 1 - 2 MB | INT8 | NPU | [FFNet-78S-Quantized.tflite](https://huggingface.co/qualcomm/FFNet-78S-Quantized/blob/main/FFNet-78S-Quantized.tflite) |
| FFNet-78S-Quantized | SA8255 (Proxy) | SA8255P Proxy | TFLITE | 5.752 ms | 1 - 3 MB | INT8 | NPU | [FFNet-78S-Quantized.tflite](https://huggingface.co/qualcomm/FFNet-78S-Quantized/blob/main/FFNet-78S-Quantized.tflite) |
| FFNet-78S-Quantized | SA8775 (Proxy) | SA8775P Proxy | TFLITE | 5.787 ms | 0 - 2 MB | INT8 | NPU | [FFNet-78S-Quantized.tflite](https://huggingface.co/qualcomm/FFNet-78S-Quantized/blob/main/FFNet-78S-Quantized.tflite) |
| FFNet-78S-Quantized | SA8650 (Proxy) | SA8650P Proxy | TFLITE | 5.717 ms | 1 - 3 MB | INT8 | NPU | [FFNet-78S-Quantized.tflite](https://huggingface.co/qualcomm/FFNet-78S-Quantized/blob/main/FFNet-78S-Quantized.tflite) |
| FFNet-78S-Quantized | QCS8450 (Proxy) | QCS8450 Proxy | TFLITE | 7.035 ms | 1 - 90 MB | INT8 | NPU | [FFNet-78S-Quantized.tflite](https://huggingface.co/qualcomm/FFNet-78S-Quantized/blob/main/FFNet-78S-Quantized.tflite) |
| FFNet-78S-Quantized | Snapdragon 8 Elite QRD | Snapdragon® 8 Elite | TFLITE | 3.501 ms | 1 - 38 MB | INT8 | NPU | [FFNet-78S-Quantized.tflite](https://huggingface.co/qualcomm/FFNet-78S-Quantized/blob/main/FFNet-78S-Quantized.tflite) |
| FFNet-78S-Quantized | Snapdragon 8 Elite QRD | Snapdragon® 8 Elite | ONNX | 8.055 ms | 7 - 76 MB | INT8 | NPU | [FFNet-78S-Quantized.onnx](https://huggingface.co/qualcomm/FFNet-78S-Quantized/blob/main/FFNet-78S-Quantized.onnx) |
| FFNet-78S-Quantized | Snapdragon X Elite CRD | Snapdragon® X Elite | ONNX | 12.351 ms | 22 - 22 MB | INT8 | NPU | [FFNet-78S-Quantized.onnx](https://huggingface.co/qualcomm/FFNet-78S-Quantized/blob/main/FFNet-78S-Quantized.onnx) |
## Installation
This model can be installed as a Python package via pip.
```bash
pip install "qai-hub-models[ffnet_78s_quantized]"
```
## Configure Qualcomm® AI Hub to run this model on a cloud-hosted device
Sign-in to [Qualcomm® AI Hub](https://app.aihub.qualcomm.com/) with your
Qualcomm® ID. Once signed in navigate to `Account -> Settings -> API Token`.
With this API token, you can configure your client to run models on the cloud
hosted devices.
```bash
qai-hub configure --api_token API_TOKEN
```
Navigate to [docs](https://app.aihub.qualcomm.com/docs/) for more information.
## Demo off target
The package contains a simple end-to-end demo that downloads pre-trained
weights and runs this model on a sample input.
```bash
python -m qai_hub_models.models.ffnet_78s_quantized.demo
```
The above demo runs a reference implementation of pre-processing, model
inference, and post processing.
**NOTE**: If you want running in a Jupyter Notebook or Google Colab like
environment, please add the following to your cell (instead of the above).
```
%run -m qai_hub_models.models.ffnet_78s_quantized.demo
```
### Run model on a cloud-hosted device
In addition to the demo, you can also run the model on a cloud-hosted Qualcomm®
device. This script does the following:
* Performance check on-device on a cloud-hosted device
* Downloads compiled assets that can be deployed on-device for Android.
* Accuracy check between PyTorch and on-device outputs.
```bash
python -m qai_hub_models.models.ffnet_78s_quantized.export
```
```
Profiling Results
------------------------------------------------------------
FFNet-78S-Quantized
Device : Samsung Galaxy S23 (13)
Runtime : TFLITE
Estimated inference time (ms) : 5.7
Estimated peak memory usage (MB): [0, 2]
Total # Ops : 156
Compute Unit(s) : NPU (156 ops)
```
## Deploying compiled model to Android
The models can be deployed using multiple runtimes:
- TensorFlow Lite (`.tflite` export): [This
tutorial](https://www.tensorflow.org/lite/android/quickstart) provides a
guide to deploy the .tflite model in an Android application.
- QNN (`.so` export ): This [sample
app](https://docs.qualcomm.com/bundle/publicresource/topics/80-63442-50/sample_app.html)
provides instructions on how to use the `.so` shared library in an Android application.
## View on Qualcomm® AI Hub
Get more details on FFNet-78S-Quantized's performance across various devices [here](https://aihub.qualcomm.com/models/ffnet_78s_quantized).
Explore all available models on [Qualcomm® AI Hub](https://aihub.qualcomm.com/)
## License
* The license for the original implementation of FFNet-78S-Quantized can be found [here](https://github.com/Qualcomm-AI-research/FFNet/blob/master/LICENSE).
* The license for the compiled assets for on-device deployment can be found [here](https://qaihub-public-assets.s3.us-west-2.amazonaws.com/qai-hub-models/Qualcomm+AI+Hub+Proprietary+License.pdf)
## References
* [Simple and Efficient Architectures for Semantic Segmentation](https://arxiv.org/abs/2206.08236)
* [Source Model Implementation](https://github.com/Qualcomm-AI-research/FFNet)
## Community
* Join [our AI Hub Slack community](https://aihub.qualcomm.com/community/slack) to collaborate, post questions and learn more about on-device AI.
* For questions or feedback please [reach out to us](mailto:ai-hub-support@qti.qualcomm.com).
|