GPTQ-for-LLaMA
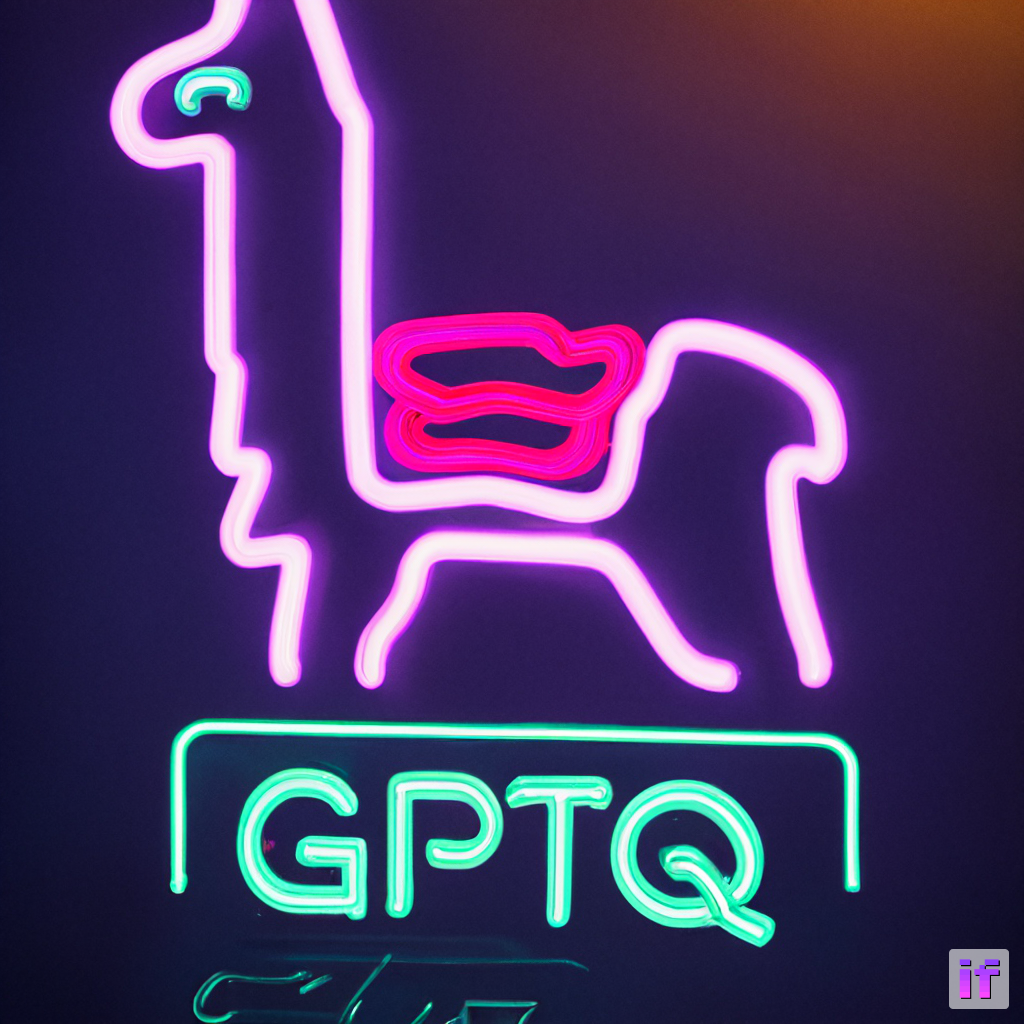
4 bits quantization of LLaMA using GPTQ
GPTQ is SOTA one-shot weight quantization method
It can be used universally, but it is not the fastest and only supports linux.
Triton only supports Linux, so if you are a Windows user, please use WSL2.
News or Update
AutoGPTQ-triton, a packaged version of GPTQ with triton, has been integrated into AutoGPTQ.
Result
LLaMA-7B(click me)
LLaMA-13B
LLaMA-33B
LLaMA-65B
Quantization requires a large amount of CPU memory. However, the memory required can be reduced by using swap memory.
Depending on the GPUs/drivers, there may be a difference in performance, which decreases as the model size increases.(https://github.com/IST-DASLab/gptq/issues/1)
According to GPTQ paper, As the size of the model increases, the difference in performance between FP16 and GPTQ decreases.
Installation
If you don't have conda, install it first.
conda create --name gptq python=3.9 -y
conda activate gptq
conda install pytorch torchvision torchaudio pytorch-cuda=11.7 -c pytorch -c nvidia
# Or, if you're having trouble with conda, use pip with python3.9:
# pip3 install torch torchvision torchaudio
git clone https://github.com/qwopqwop200/GPTQ-for-LLaMa
cd GPTQ-for-LLaMa
pip install -r requirements.txt
Dependencies
torch
: tested on v2.0.0+cu117transformers
: tested on v4.28.0.dev0datasets
: tested on v2.10.1safetensors
: tested on v0.3.0
All experiments were run on a single NVIDIA RTX3090.
Language Generation
LLaMA
#convert LLaMA to hf
python convert_llama_weights_to_hf.py --input_dir /path/to/downloaded/llama/weights --model_size 7B --output_dir ./llama-hf
# Benchmark language generation with 4-bit LLaMA-7B:
# Save compressed model
CUDA_VISIBLE_DEVICES=0 python llama.py ${MODEL_DIR} c4 --wbits 4 --true-sequential --act-order --groupsize 128 --save llama7b-4bit-128g.pt
# Or save compressed `.safetensors` model
CUDA_VISIBLE_DEVICES=0 python llama.py ${MODEL_DIR} c4 --wbits 4 --true-sequential --act-order --groupsize 128 --save_safetensors llama7b-4bit-128g.safetensors
# Benchmark generating a 2048 token sequence with the saved model
CUDA_VISIBLE_DEVICES=0 python llama.py ${MODEL_DIR} c4 --wbits 4 --groupsize 128 --load llama7b-4bit-128g.pt --benchmark 2048 --check
# Benchmark FP16 baseline, note that the model will be split across all listed GPUs
CUDA_VISIBLE_DEVICES=0,1,2,3,4 python llama.py ${MODEL_DIR} c4 --benchmark 2048 --check
# model inference with the saved model
CUDA_VISIBLE_DEVICES=0 python llama_inference.py ${MODEL_DIR} --wbits 4 --groupsize 128 --load llama7b-4bit-128g.pt --text "this is llama"
# model inference with the saved model using safetensors loaded direct to gpu
CUDA_VISIBLE_DEVICES=0 python llama_inference.py ${MODEL_DIR} --wbits 4 --groupsize 128 --load llama7b-4bit-128g.safetensors --text "this is llama" --device=0
# model inference with the saved model with offload(This is very slow).
CUDA_VISIBLE_DEVICES=0 python llama_inference_offload.py ${MODEL_DIR} --wbits 4 --groupsize 128 --load llama7b-4bit-128g.pt --text "this is llama" --pre_layer 16
It takes about 180 seconds to generate 45 tokens(5->50 tokens) on single RTX3090 based on LLaMa-65B. pre_layer is set to 50.
Basically, 4-bit quantization and 128 groupsize are recommended.
You can also export quantization parameters with toml+numpy format.
CUDA_VISIBLE_DEVICES=0 python llama.py ${MODEL_DIR} c4 --wbits 4 --true-sequential --act-order --groupsize 128 --quant-directory ${TOML_DIR}
Acknowledgements
This code is based on GPTQ
Thanks to Meta AI for releasing LLaMA, a powerful LLM.
Triton GPTQ kernel code is based on GPTQ-triton