license: apache-2.0
language:
- vi
- en
- zh
base_model:
- Qwen/Qwen2-VL-2B-Instruct
library_name: transformers
tags:
- erax
- multimodal
- erax-vl-2B
- insurance
- ocr
- vietnamese
- bcg
pipeline_tag: visual-question-answering
widget:
- src: images/photo-1-16505057982762025719470.webp
example_title: Test 1
- src: images/vt-don-thuoc-f0-7417.jpeg
example_title: Test 2
EraX-VL-7B-V1.5
Introduction 🎉
Hot on the heels of the popular EraX-VL-7B-V1.0 model, we proudly present EraX-VL-7B-V1.5, another robust multimodal model for OCR (optical character recognition) and VQA (visual question-answering) that excels in various languages 🌍, with a particular focus on Vietnamese 🇻🇳. This model stands out for its precise recognition capabilities across a range of documents 📝, including medical forms 🩺, invoices 🧾, bills of sale 💳, quotes 📄, and medical records 💊. This functionality is expected to be highly beneficial for hospitals 🏥, clinics 💉, insurance companies 🛡️, and other similar applications 📋. Built on the solid foundation of the Qwen/Qwen2-VL-2B-Instruct[1], which we found to be of high quality and fluent in Vietnamese, EraX-VL-7B-V1.5
has been fine-tuned to enhance its performance. We plan to continue improving and releasing new versions for free, along with sharing performance benchmarks in the near future.
One standing-out feature of EraX-VL-7B-V1.5 is the capability to do multi-turn Q&A with impressive reasoning capability!
NOTA BENE:
- EraX-VL-7B-V1.5 is NOT a typical OCR-only tool likes Tesseract but is a Multimodal LLM-based model. To use it effectively, you may have to twist your prompt carefully depending on your tasks.
- This model was NOT finetuned with medical (X-ray) dataset or car accidences (yet). Stay tune for updated version coming up sometime early 2025.
EraX-VL-7B-V1.5 is a young member of our EraX's LànhGPT collection of LLM models.
- Developed by:
- Nguyễn Anh Nguyên (nguyen@erax.ai)
- Nguyễn Hồ Nam (BCG)
- Phạm Huỳnh Nhật (nhat.ph@erax.ai)
- Phạm Đình Thục (thuc.pd@erax.ai)
- Funded by: Bamboo Capital Group and EraX
- Model type: Multimodal Transformer with over 7B parameters
- Languages (NLP): Primarily Vietnamese with multilingual capabilities
- License: Apache 2.0
- Fine-tuned from: Qwen/Qwen2-VL-7B-Instruct
- Prompt examples: Some popular prompt examples on Github.
Benchmarks 📊
🏆 LeaderBoard
The EraX-VL-7B-V1.5 achieved exceptionally high performance compared to other models of equal size or even 10 times larger, and we open-source! You can re-run the benchmark at any time.
Models | Open-Source | VI-MTVQA |
---|---|---|
EraX-VL-7B-V1.5 🥇 | ✅ | 47.2 |
Qwen2-VL 72B 🥈 | ✘ | 41.6 |
ViGPT-VL 🥉 | ✘ | 39.1 |
EraX-VL-2B-V1.5 | ✅ | 38.2 |
EraX-VL-7B-V1 | ✅ | 37.6 |
Vintern-1B-V2 | ✅ | 37.4 |
Qwen2-VL 7B | ✅ | 30.0 |
Claude3 Opus | ✘ | 29.1 |
GPT-4o mini | ✘ | 29.1 |
GPT-4V | ✘ | 28.9 |
Gemini Ultra | ✘ | 28.6 |
InternVL2 76B | ✅ | 26.9 |
QwenVL Max | ✘ | 23.5 |
Claude3 Sonnet | ✘ | 20.8 |
QwenVL Plus | ✘ | 18.1 |
MiniCPM-V2.5 | ✅ | 15.3 |
The test code for evaluating models in the paper can be found in: EraX-JS-Company/EraX-MTVQA-Benchmark
API trial 🎉
Please contact nguyen@erax.ai for API access inquiry.
Examples 🧩
1. OCR - Optical Character Recognition for Multi-Images
Example 01: Citizen identification card

Front View

Back View
Source: Google Support
{
"Số thẻ": "037094012351",
"Họ và tên": "TRỊNH QUANG DUY",
"Ngày sinh": "04/09/1994",
"Giới tính": "Nam",
"Quốc tịch": "Việt Nam",
"Quê quán": "Tân Thành, Kim Sơn, Ninh Bình",
"Nơi thường trú": "Xóm 6\nTân Thành, Kim Sơn, Ninh Bình",
"Có giá trị đến": "04/09/2034",
"Đặc điểm nhân dạng": "sẹo chấm c. 1cm trên đuôi mắt trái",
"Nơi cấp": "CỤC TRƯỞNG CỤC CẢNH SÁT\nQUẢN LÝ HÀNH CHÍNH VỀ TRẬT TỰ XÃ HỘI",
"Ngày cấp": "10/12/2022",
"Cán bộ ký tên": "Nguyễn Quốc Hùng",
"Mã định danh": "IDVNM0940123513037094012351"
}
Example 02: Driver's License
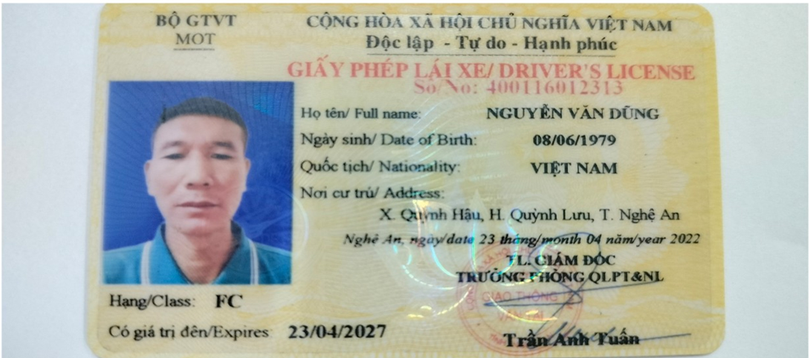
Front View
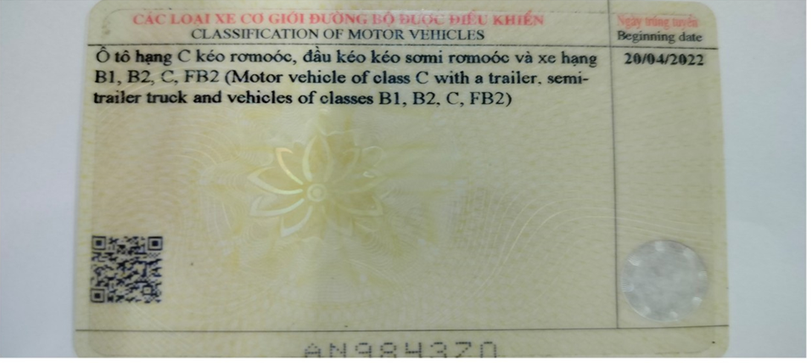
Back View
Source: Báo Pháp luật
{
"No.":"400116012313"
"Fullname":"NGUYỄN VĂN DŨNG"
"Date_of_birth":"08/06/1979"
"Nationality":"VIỆT NAM"
"Address":"X. Quỳnh Hầu, H. Quỳnh Lưu, T. Nghệ An
Nghệ An, ngày/date 23 tháng/month 04 năm/year 2022"
"Hang_Class":"FC"
"Expires":"23/04/2027"
"Place_of_issue":"Nghệ An"
"Date_of_issue":"ngày/date 23 tháng/month 04 năm/year 2022"
"Signer":"Trần Anh Tuấn"
"Các loại xe được phép":"Ô tô hạng C kéo rơmoóc, đầu kéo kéo sơmi rơmoóc và xe hạng B1, B2, C, FB2 (Motor vehicle of class C with a trailer, semi-trailer truck and vehicles of classes B1, B2, C, FB2)"
"Mã số":""
}
Example 03: Vehicle Registration Certificate
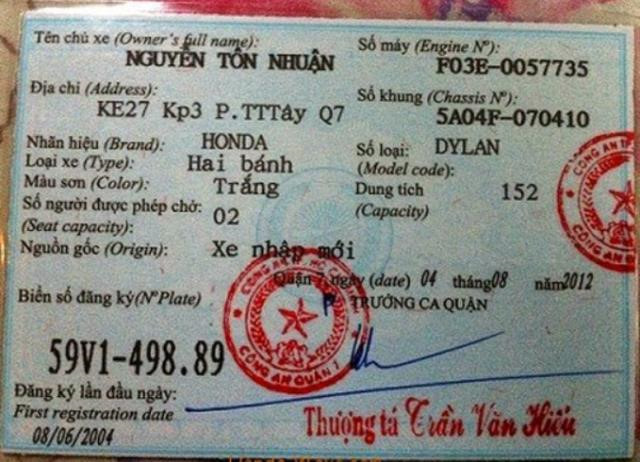
Source: Báo Vietnamnet
{
"Tên chủ xe": "NGUYỄN TÔN NHUẬN",
"Địa chỉ": "KE27 Kp3 P.TTTây Q7",
"Nhãn hiệu": "HONDA",
"Số loại": "DYLAN",
"Màu sơn": "Trắng",
"Năm sản xuất": "2012",
"Số máy": "F03E-0057735",
"Số khung": "SA04F-070410",
"Dung tích": "152",
"Số chỗ ngồi": "02",
"Biển số đăng ký": "59V1-498.89",
"Đăng ký lần đầu ngày": "08/06/2004",
"Chức vụ": "Thượng tá",
"Người ký": "Trần Văn Hiểu"
}
Example 04: Vehicle Registration

Source: https://llumar.com.vn
{
"vehicle": {
"registration_number": "30A-072.36",
"vehicle_inspection_number": "2903V-093515",
"type": "ô tô con",
"mark": "MERCEDES-BENZ",
"model_code": "C300 W204",
"engine_number": "27294732096079",
"chassis_number": "RLMGF5EX3DV005333",
"manufactured_year_and_country": "2013, Việt Nam",
"life_time_limit_to": "",
"commercial_use": "",
"modification": ""
},
"specifications": {
"wheel_formula": "4x2",
"wheel_tread": "1521/1512 (mm)",
"overall_dimension": "4650 x 1770 x 1429 (mm)",
"largest_luggage_container_dimension": "",
"wheelbase": "2760 (mm)",
"kerb_mass": "1575 (kg)",
"design_authorized_pay_load": "",
"design_authorized_total_mass": "2090/2090 (kg)",
"design_authorized_towed_mass": "",
"permissible_number_of_pers_carried": "5 chỗ ngồi, 0 chỗ đứng, 0 chỗ nằm",
"type_of_fuel_used": "Xăng",
"engine_displacement": "2996 (cm3)",
"max_output_per_rpm": "170(kW)/6000vph",
"number": "KC-1292285"
},
"inspection_report_number": "2905V-20953/16",
"valid_until": "31/01/2018",
"place_date_of_issue": "Hà Nội, ngày 1 tháng 8 năm 2016",
"inspection_center": "ĐƠN VỊ KIỂM ĐỊNH XE CƠ GIỚI",
"signature": "Ngọc Tuấn",
"equipped_with_tachograph": "",
"inspection_stamp_was_not_issued": "",
"notes": "Biển đăng ký nền trắng"
}
Example 05: Receipt

Source: https://tintucketoan.com/
{
'Mẫu số': '01GKTKT3/001',
'Ký hiệu': 'TC/18P',
'Số': '0000030',
'Họ tên người mua hàng': None,
'Tên đơn vị': 'Công Ty TNHH Kế Toán Hà Nội',
'Mã số thuế': '0106235869',
'Địa chỉ': 'Số 49 Ngõ 322 Lê Trọng Tấn, phường Khương Mai, quận Thanh Xuân, Hà Nội',
'Hình thức thanh toán': 'TM',
'STT': None,
'Tên hàng hóa, dịch vụ': 'Tra cứu phần mềm thư viện pháp luật trực tuyến',
'Đơn vị tính': None,
'Số lượng': None,
'Đơn giá': '168.000',
'Thành tiền': '2.016.000',
'Thuế suất GTGT': None,
'Tiền thuế GTGT': None,
'Tổng cộng tiền thanh toán': '2.016.000',
'Số tiền viết bằng chữ': 'Hai triệu, không trăm mười sáu nghìn đồng',
'Người bán hàng': 'Bùi Văn Hùng',
'Chức vụ người bán hàng': 'TRƯỞNG CHI NHÁNH'
}
2.1 Image Captioning

Hình ảnh là biểu đồ BMI theo tuổi, thể hiện mối quan hệ giữa chỉ số khối cơ thể (BMI) và độ tuổi của trẻ em. Biểu đồ được chia thành các vùng màu khác nhau tương ứng với các mức BMI khác nhau:
- Vùng màu đỏ: Chỉ số BMI cao hơn 25, cho thấy tình trạng béo phì.
- Vùng màu vàng: Chỉ số BMI nằm trong khoảng từ 18 đến 25, cho thấy nguy cơ béo phì.
- Vùng màu xanh lá cây nhạt: Chỉ số BMI nằm trong khoảng từ 16 đến 18, cho thấy sức khỏe dinh dưỡng tốt.
- Vùng màu xanh lá cây đậm: Chỉ số BMI thấp hơn 16, cho thấy tình trạng thiếu cân.
Trục tung biểu diễn chỉ số BMI, trục hoành biểu diễn tuổi (tính bằng năm). Đường cong màu xám đậm thể hiện đường chuẩn BMI theo tuổi. Các đường cong này cho thấy sự thay đổi BMI theo thời gian, giúp đánh giá sự phát triển cân nặng của trẻ em. Ví dụ, ở trẻ em dưới 3 tuổi, BMI thường dao động trong vùng thiếu cân hoặc sức khỏe dinh dưỡng tốt. Khi trẻ lớn lên, BMI có xu hướng tăng dần, nhưng tốc độ tăng trưởng có thể khác nhau tùy thuộc vào từng cá nhân. Biểu đồ cũng hiển thị các phần trăm phân vị (Percentile), cho biết tỷ lệ phần trăm trẻ em có BMI thấp hơn hoặc cao hơn so với một nhóm trẻ em cùng độ tuổi. Điều này giúp so sánh BMI của trẻ em với tiêu chuẩn quốc tế.
2.2 Image Captioning
.jpg)
Hình ảnh chụp một cảnh tượng đầy xúc động và bi thảm, dường như diễn ra ở một khu vực nghèo khó, có thể là một khu định cư hoặc khu ổ chuột. Trung tâm của bức ảnh là một chiếc xe đẩy được kéo bởi một con lừa. Trên xe đẩy có một nhóm người, bao gồm một người đàn ông lớn tuổi có vẻ như là người hướng dẫn, một phụ nữ mặc áo choàng đen, một phụ nữ trẻ mặc áo xám, một bé gái nhỏ được che mặt bằng khăn trùm đầu, và một cậu bé mặc áo xanh lá cây. Họ có vẻ như đang di chuyển từ một khu vực bị tàn phá sang một nơi khác. Xung quanh họ là những mảnh vụn, rác thải và những tòa nhà đổ nát, cho thấy một môi trường sống khó khăn và thiếu thốn. Phía sau họ, có một số người khác đang đi bộ hoặc đi xe đạp, một số người đang mang theo hành lý. Bầu trời trong xanh, nhưng không khí chung của bức ảnh là nặng nề và buồn bã. Có thể đây là một cảnh tượng di cư, tị nạn, hoặc một sự kiện thảm khốc nào đó.
3. Multi-turns Visual Question Answering and Reasoning

Source: https://kenh14.vn
User: Hãy cho biết thông tin bệnh nhân
Assistant: Tên: NGUYỄN THỊ THÁI TRINH, Ngày sinh: 09/09/1993, Giới tính: Nữ, Địa chỉ: TÂN ĐỊNH - Q1 - TPHCM, Mã thẻ BHYT: 19169080, Số khám bệnh: 2569501, Mã khu vực (K1/K2/K3): 2
User: Họ được chuẩn đoán bị bệnh gì?
Assistant: Nhiễm trùng đường ruột do vi khuẩn, không xác định loài
User: Người bệnh có tiền sử bệnh không? Nếu có thì là gì?
Assistant: Thiếu Calci do chế độ ăn
User: Bệnh nhân đã thực hiện những xét nghiệm gì?
Assistant: [NQ286] Tổng phân tích tế bào máu ngoại vi (bằng máy đếm laser), [NQ286] Điện giải đồ (Na, K, Cl) [Máu], [NQ286] Đo hoạt độ ALT (GPT) [Máu], [NQ286] Đo hoạt độ AST (GOT) [Máu], [NQ286] Định lượng Calci toàn phần [Máu], [NQ286] Định lượng Creatinin [Máu], [NQ286] Định lượng Glucose [Máu], [NQ286] Định lượng Urê máu [Máu], [NQ286] Siêu âm ổ bụng (gan mật, tụy, lách, thận, bàng quang)
User: Số tiền Vật tư y tế mà người bệnh phải trả là bao nhiêu?
Assistant: 19,708
User: Các loại thuốc dịch truyền người bệnh phải trả là gì, tổng số tiền là bao nhiêu?
Assistant: Atropin Sulfat (Syv2019), Bicefzidim 1g (Syv2019), GONCAL (Syv2019), Lactated Ringer's-500ml (Syv2019), Nước cất pha tiêm 5ml (Syv2019), Sodium Chloride 0.9% -500ml (Syv2019), Vincomid (Syv2019), Vinopa (Syv2019), tổng cộng 45,234 đồng
Quickstart 🎮
Install the necessary packages:
python -m pip install git+https://github.com/huggingface/transformers accelerate
python -m pip install qwen-vl-utils
pip install flash-attn --no-build-isolation
Then you can use EraX-VL-7B-V1.5
like this:
import os
import base64
import json
import cv2
import numpy as np
import matplotlib.pyplot as plt
import torch
from transformers import Qwen2VLForConditionalGeneration, AutoTokenizer, AutoProcessor
from qwen_vl_utils import process_vision_info
model_path = "erax/EraX-VL-7B-V1.5"
model = Qwen2VLForConditionalGeneration.from_pretrained(
model_path,
torch_dtype=torch.bfloat16,
attn_implementation="eager", # replace with "flash_attention_2" if your GPU is Ampere architecture
device_map="auto"
)
tokenizer = AutoTokenizer.from_pretrained(model_path)
# processor = AutoProcessor.from_pretrained(model_path)
min_pixels = 256 * 28 * 28
max_pixels = 1280 * 28 * 28
processor = AutoProcessor.from_pretrained(
model_path,
min_pixels=min_pixels,
max_pixels=max_pixels,
)
image_path ="image.jpg"
with open(image_path, "rb") as f:
encoded_image = base64.b64encode(f.read())
decoded_image_text = encoded_image.decode('utf-8')
base64_data = f"data:image;base64,{decoded_image_text}"
messages = [
{
"role": "user",
"content": [
{
"type": "image",
"image": base64_data,
},
{
"type": "text",
"text": "Trích xuất thông tin nội dung từ hình ảnh được cung cấp."
},
],
}
]
# Prepare prompt
tokenized_text = processor.apply_chat_template(
messages, tokenize=False, add_generation_prompt=True
)
image_inputs, video_inputs = process_vision_info(messages)
inputs = processor(
text=[ tokenized_text],
images=image_inputs,
videos=video_inputs,
padding=True,
return_tensors="pt",
)
inputs = inputs.to("cuda")
# Generation configs
generation_config = model.generation_config
generation_config.do_sample = True
generation_config.temperature = 1.0
generation_config.top_k = 1
generation_config.top_p = 0.9
generation_config.min_p = 0.1
generation_config.best_of = 5
generation_config.max_new_tokens = 2048
generation_config.repetition_penalty = 1.06
# Inference
generated_ids = model.generate(**inputs, generation_config=generation_config)
generated_ids_trimmed = [
out_ids[len(in_ids) :] for in_ids, out_ids in zip(inputs.input_ids, generated_ids)
]
output_text = processor.batch_decode(
generated_ids_trimmed, skip_special_tokens=True, clean_up_tokenization_spaces=False
)
print(output_text[0])
References 📑
[1] Qwen team. Qwen2-VL. 2024.
[2] Bai, Jinze, et al. "Qwen-VL: A Versatile Vision-Language Model for Understanding, Localization, Text Reading, and Beyond." arXiv preprint arXiv:2308.12966 (2023).
[4] Yang, An, et al. "Qwen2 technical report." arXiv preprint arXiv:2407.10671 (2024).
[5] Chen, Zhe, et al. "Internvl: Scaling up vision foundation models and aligning for generic visual-linguistic tasks." Proceedings of the IEEE/CVF Conference on Computer Vision and Pattern Recognition. 2024.
[6] Chen, Zhe, et al. "How far are we to gpt-4v? closing the gap to commercial multimodal models with open-source suites." arXiv preprint arXiv:2404.16821 (2024).
[7] Tran, Chi, and Huong Le Thanh. "LaVy: Vietnamese Multimodal Large Language Model." arXiv preprint arXiv:2404.07922 (2024).
Contact 🤝
- For correspondence regarding this work or inquiry for API trial, please contact Nguyễn Anh Nguyên at nguyen@erax.ai.
- Follow us on EraX Github